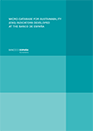
Series: Statistical Notes. 18.
Author: Alejandro Morales Fernández.
Full document
Summary
This statistical note presents the work carried out last year by the Banco de España’s Central Balance Sheet Data Office (CBSO) on the sectorisation and classification of holding companies using machine learning. This work has also been presented, in July 2023, at the World Statistics Congress (WSC) in Ottawa, organised by the International Statistics Institute (ISI), and this note is part of a series of talks on central banks organised by the Irving Fisher Committee (IFC) at the same congress.
The work presented can be divided into two parts: first, obtaining an automated procedure to help distinguish companies that, given their economic activity, are either holding companies or head offices. In other words, the aim of this work is to detect companies whose activities may come under codes 6420 or 7010 of the CNAE (Spanish National Classification of Economic Activities, equivalent to NACE, the statistical classification of economic activities in the European Community), by checking whether their data (mainly economic and financial ratios from their annual financial statements) suggest that they are or may be holding companies or head offices (whether or not they report such activities). The second part of the work is the classification of holding companies and head offices into the financial or non-financial sectors (as required by the National Accounts), using the model and information generated by the first part of the project as a starting point.
Artificial intelligence – in particular supervised machine learning classification models – is used to perform both of these tasks. A supervised model requires a prior set of labelled companies, that is to say it needs companies that have already been categorised with complete certainty as holding companies, head offices or other companies in the financial or non-financial sectors. A wide range of companies in the databases of the CBSO Division of the Statistics Department have been categorised manually, so that labelled information – an essential factor for building the model – is available.
Other essential tasks for the creation of the final machine learning model have also been performed, including the integration of various CBSO data sources and their subsequent adaptation to the structure necessary to create the model. Inter alia, variables have been selected, eliminated and transformed using statistical methods, and variables have been selected and/or eliminated for business reasons.
Finally, after the model has been constructed and evaluated, a quality control procedure is proposed. The proposed CNAE codes sometimes differ from those originally recorded. In such cases, two independent actions are proposed as a result of the model’s application: the automatic classification of over 8,500 companies, where the model’s result is in line with the business rules, and the manual review of approximately 5,300 other companies. As for the institutional sectorisation model, it provides a smaller set of entities for which the sector needs to be reviewed and therefore saves human effort.
The steps taken to build the proposed model, along with other technical details, are described in the annex on the technical details of the model.